Hugging Face Improves Compute Scaling
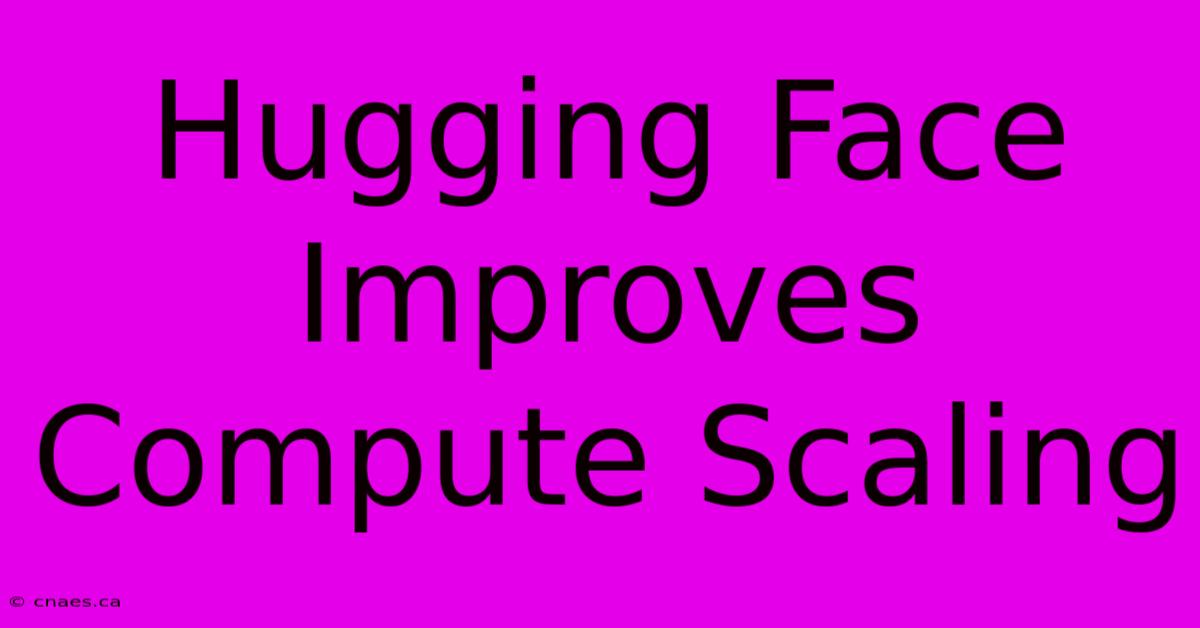
Discover more detailed and exciting information on our website. Click the link below to start your adventure: Visit My Website. Don't miss out!
Table of Contents
Hugging Face Improves Compute Scaling: A Game Changer for Machine Learning
Hugging Face, the renowned platform for all things machine learning (ML), has significantly improved its compute scaling capabilities. This upgrade is a game-changer for researchers and developers, offering enhanced efficiency and accessibility to powerful computational resources. This article delves into the specifics of these advancements and explores their implications for the broader ML community.
Faster Training, Bigger Models
Previously, scaling compute resources on Hugging Face for large-scale model training could be a complex and time-consuming process. The improved infrastructure now allows for much faster and more seamless scaling. This means researchers can train larger and more complex models in significantly less time. This reduction in training time translates directly into faster iteration cycles, allowing for quicker experimentation and ultimately, faster progress in ML research and development.
Benefits of Enhanced Scaling:
- Reduced Training Time: The most significant benefit is the dramatic reduction in the time required to train models. This is particularly crucial for computationally intensive tasks like training large language models (LLMs).
- Increased Model Complexity: Researchers can now explore more complex models with a larger number of parameters, leading to potentially more accurate and powerful AI systems.
- Improved Accessibility: The simplified scaling process makes it easier for individuals and smaller teams to access the computational resources they need, democratizing access to powerful ML tools.
- Cost Optimization: While increased compute power might seem expensive, the speed improvements can often lead to overall cost savings by reducing the total time spent on training.
Impact on the ML Community
The improved compute scaling from Hugging Face has widespread implications for the entire ML community:
- Accelerated Research: Faster training enables researchers to explore new ideas and algorithms more quickly, pushing the boundaries of what's possible in AI.
- Increased Innovation: Easier access to computational resources empowers a broader range of individuals and organizations to contribute to the field, fostering a more diverse and innovative landscape.
- Wider Adoption of ML: The simplified process encourages greater adoption of ML technologies across various industries, accelerating the integration of AI into everyday applications.
Future Implications and Expectations
Hugging Face's commitment to improving its infrastructure suggests a continued focus on making ML more accessible and efficient. We can expect further advancements in compute scaling and other areas, making the platform even more powerful and user-friendly. This ongoing development will likely drive further innovation and democratization within the field of machine learning.
Conclusion
Hugging Face's improvements to compute scaling represent a substantial leap forward for the ML community. By accelerating training times, increasing accessibility, and lowering barriers to entry, this enhancement empowers researchers and developers to achieve more, faster. The impact of these advancements will undoubtedly be felt across various sectors, driving further innovation and progress in artificial intelligence. This is a significant step towards making cutting-edge machine learning technology available to a wider audience.
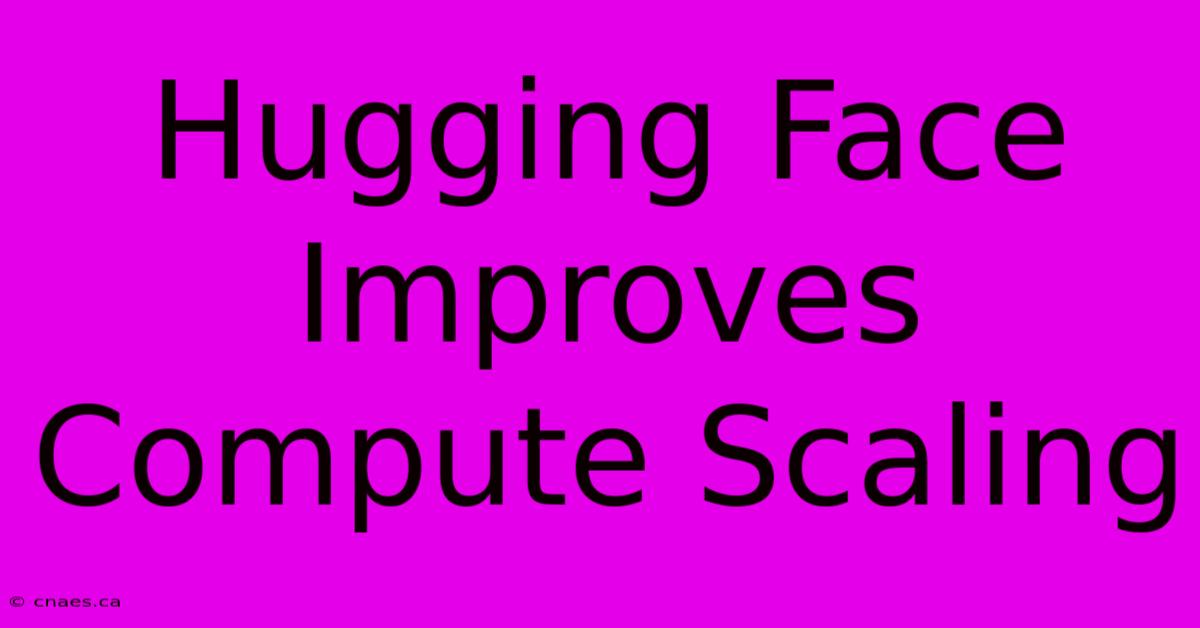
Thank you for visiting our website wich cover about Hugging Face Improves Compute Scaling. We hope the information provided has been useful to you. Feel free to contact us if you have any questions or need further assistance. See you next time and dont miss to bookmark.
Also read the following articles
Article Title | Date |
---|---|
Mexican Retail In Nordstrom Family Acquisition | Dec 24, 2024 |
Open Christmas Eve 2024 Guide | Dec 24, 2024 |
Christmas Day P E I Holiday Hours Guide | Dec 24, 2024 |
Womens Odi India Vs Wi Highlights | Dec 24, 2024 |
Serie A Referee Faces Backlash | Dec 24, 2024 |