Free AI Data: Harvard Funding
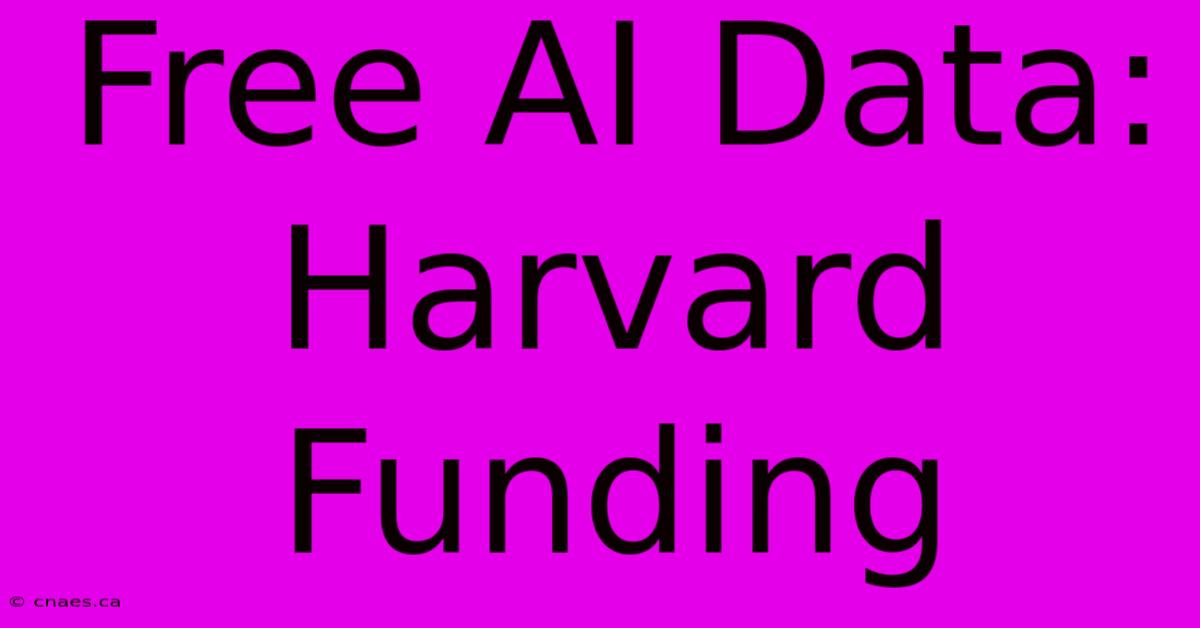
Discover more detailed and exciting information on our website. Click the link below to start your adventure: Visit My Website. Don't miss out!
Table of Contents
Free AI Data: Harvard Funding โ A Boon for Researchers?
The world of artificial intelligence (AI) is data-hungry. Vast datasets are crucial for training sophisticated AI models, but acquiring high-quality, labeled data can be incredibly expensive and time-consuming. This is where initiatives like Harvard's funding for free AI data become incredibly significant. This article explores the implications of Harvard's commitment to making AI datasets freely available, examining its potential benefits and challenges.
Harvard's Commitment to Open AI Data
Harvard University, a global leader in research and education, recognizes the importance of open access to data for advancing AI research. While the specifics of their funding may vary across different projects and departments, their overall commitment points towards a future where valuable datasets aren't locked behind paywalls. This initiative directly addresses a major bottleneck in AI development, allowing researchers, especially those with limited resources, to participate more fully in the field.
Benefits of Free AI Data from Harvard (and Similar Initiatives):
- Increased Accessibility: The most obvious benefit is the democratization of AI research. Researchers at smaller institutions, in developing countries, or with limited budgets can now access high-quality data they might otherwise be unable to afford. This fosters a more inclusive and diverse AI community.
- Faster Innovation: Broader access to data accelerates the pace of innovation. More researchers working with the same datasets can identify patterns, develop new algorithms, and ultimately push the boundaries of AI capabilities more quickly.
- Improved Reproducibility: Open datasets promote reproducibility, a crucial aspect of scientific rigor. Researchers can replicate experiments, verify results, and build upon existing work, enhancing the reliability and trustworthiness of AI research findings.
- Collaboration and Knowledge Sharing: Free data encourages collaboration. Researchers can share their findings and insights, fostering a collaborative environment that leads to more robust and impactful AI advancements.
- Addressing Bias: Open datasets allow for scrutiny and identification of biases present in data. By openly sharing and analyzing these datasets, the AI community can work collectively to mitigate biases and create more equitable AI systems.
Challenges and Considerations
Despite the numerous advantages, several challenges need to be addressed:
- Data Quality and Annotation: Ensuring the quality and accuracy of freely available data is paramount. Poorly annotated or inaccurate data can lead to flawed AI models and unreliable results. Rigorous quality control mechanisms are essential.
- Data Privacy and Ethical Concerns: When dealing with sensitive information, ensuring data privacy and ethical considerations are paramount. Anonymization techniques and robust data governance policies are necessary to prevent misuse and protect individual privacy.
- Sustainability of Funding: Sustaining the funding for data collection, curation, and distribution is crucial for the long-term success of these initiatives. Securing consistent funding models is a challenge that needs ongoing attention.
- Data Governance and Intellectual Property: Clear guidelines on data usage, intellectual property rights, and attribution are needed to avoid conflicts and ensure responsible data sharing. Well-defined licensing agreements are crucial.
Conclusion: A Promising Future
Harvard's funding for free AI data represents a significant step towards a more inclusive and innovative AI ecosystem. While challenges remain, the potential benefits โ increased accessibility, faster innovation, and improved reproducibility โ outweigh the risks. As more institutions follow suit and invest in open AI data initiatives, the field will undoubtedly benefit, leading to more robust, ethical, and impactful AI technologies. The focus now should be on addressing the challenges effectively to fully realize the transformative potential of freely available datasets.
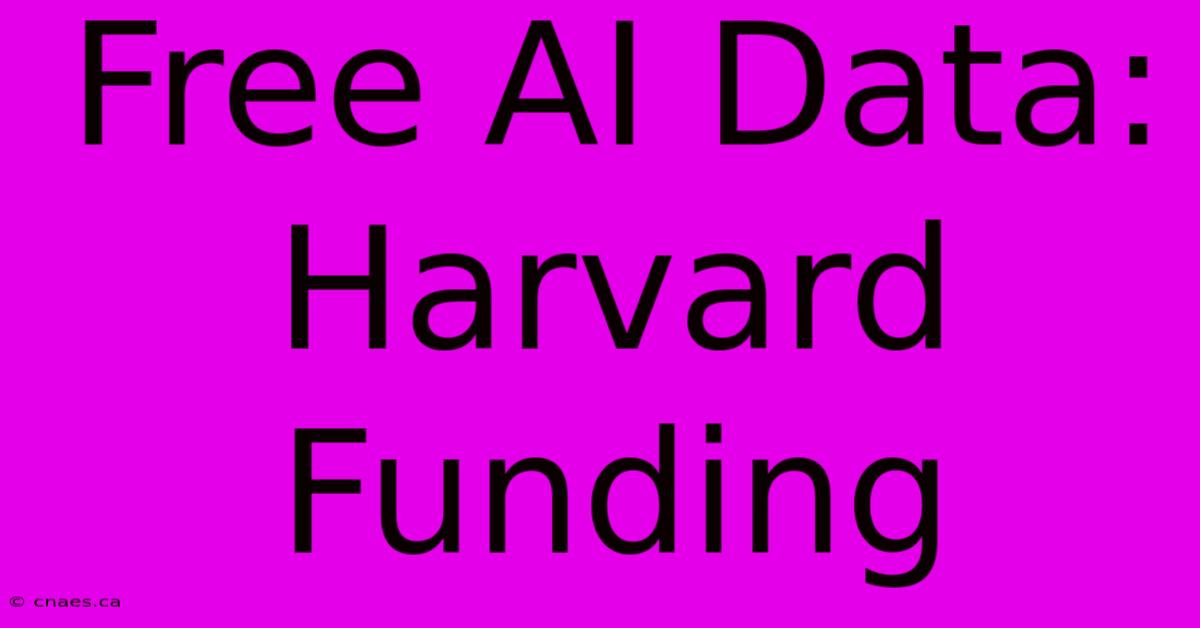
Thank you for visiting our website wich cover about Free AI Data: Harvard Funding. We hope the information provided has been useful to you. Feel free to contact us if you have any questions or need further assistance. See you next time and dont miss to bookmark.
Also read the following articles
Article Title | Date |
---|---|
Deeming Defamation Case Pesutto Loses | Dec 12, 2024 |
Trump Names Lake Voice Of Director | Dec 12, 2024 |
Googles Gemini 2 0 Conversational Ai | Dec 12, 2024 |
Arsenal 3 0 Monacos Defeat | Dec 12, 2024 |
Dortmund Barcelona Champions League Live Match | Dec 12, 2024 |